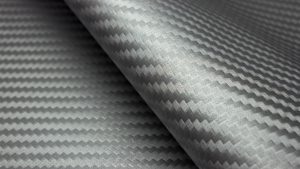
Magnetic resonance imaging (MRI) has been used clinically for many years. Image interpretation is almost always qualitative — differences in contrast between anatomical structures are used to distinguish between normal and abnormal tissues. But, from the outset, scientists and clinicians understood the potential power of quantitative MRI (qMRI), which can be used to measure a wide range of chemical, physical and physiological properties. qMRI is becoming more and more common, thanks to technological advancements overcoming some of the inherent challenges, but the uncertainty associated with the measurements obtained is rarely discussed.
This course will help MR physicists determine the measurement uncertainty associated with MRI relaxation parameters. We focus on T2 measurement throughout, but the content can also be applied to T1 measurement.
Consisting of one module of three lessons, this course will:
The course was developed for MR physicists who understand the measurement data they obtain. They may have used ‘black box’ software to fit an exponential model to that data, but have little knowledge of:
We strongly recommend you take our ‘Measurement Explained’ and ‘Metrology for MRI’ e-learning courses before this one. We also recommend ‘Uncertainty Propagation for Quantitative MRI’, ‘Introduction to Measurement Uncertainty’ and ‘Understanding Uncertainty Budgets’. Please see our course catalogue for more information about these courses.
The code included in this course has been developed for and is intended for use in a research environment only. No endorsement can be given for other use including, but not limited to, use in a clinical environment.
Privacy Policy | Cookie Policy
© NPL Management Limited 2024 | Hampton Road, Teddington, Middlesex, TW11 0LW | Tel: 020 8977 3222